Computer vision in a painting refers to the use of computer technology to analyze and interpret visual content within a painting. It involves techniques such as image processing, object detection, and scene recognition to extract meaningful information from the artwork.
This field has gained significant importance in art history, conservation, and connoisseurship. Computer vision algorithms can assist experts in tasks such as identifying the artist’s style, detecting forgeries, analyzing brushstrokes, and uncovering hidden details invisible to the naked eye. Additionally, it plays a crucial role in digitizing and preserving cultural heritage by creating high-resolution images and 3D models of paintings.
The study of computer vision in paintings has opened up new avenues for research and exploration in the art world. It has enhanced our understanding of artistic techniques, provided insights into the creative process, and contributed to the preservation and appreciation of cultural heritage.
computer vision in a painting
Computer vision in a painting encompasses various key aspects that contribute to its significance and applications. These include:
- Digitization: Converting paintings into digital formats for preservation and analysis.
- Authentication: Identifying forgeries and determining the authenticity of paintings.
- Attribution: Establishing the artist or school responsible for a particular painting.
- Style analysis: Examining brushstrokes, color palettes, and compositions to identify artistic styles.
- Damage assessment: Detecting and analyzing damage to paintings for conservation purposes.
- Hidden details: Uncovering underlying layers, sketches, or inscriptions that are invisible to the naked eye.
- 3D modeling: Creating three-dimensional representations of paintings for immersive experiences and research.
These aspects work together to enhance our understanding of paintings, facilitate art historical research, and contribute to the preservation and appreciation of cultural heritage. For example, computer vision algorithms have been used to analyze the brushstrokes of Vincent van Gogh, revealing the unique characteristics that distinguish his works from those of other artists. In another instance, computer vision techniques have helped conservators identify hidden layers in paintings, providing valuable insights into the artist’s creative process and the evolution of the artwork over time.
Digitization
Digitization is a crucial component of computer vision in a painting. By converting paintings into digital formats, we create high-resolution images that can be analyzed and processed by computer algorithms. This process enables a wide range of applications, including preservation, analysis, and research.
For preservation purposes, digitization allows us to create permanent digital records of paintings. These digital images can be stored in archives and accessed remotely, ensuring that the artwork remains accessible even if the original painting is damaged or lost. Digitization also facilitates the creation of virtual exhibitions and online galleries, making it possible for people around the world to view and appreciate paintings that may not be physically accessible to them.
In terms of analysis, digitization enables computer vision algorithms to perform detailed examinations of paintings. These algorithms can analyze brushstrokes, color palettes, and other visual features to identify patterns and characteristics that may not be visible to the naked eye. This information can be used for art historical research, attribution studies, and the detection of forgeries.
For example, computer vision algorithms have been used to analyze the brushstrokes of Vincent van Gogh, revealing the unique characteristics that distinguish his works from those of other artists. In another instance, computer vision techniques have helped conservators identify hidden layers in paintings, providing valuable insights into the artist’s creative process and the evolution of the artwork over time.
Overall, the digitization of paintings is essential for the development and application of computer vision in a painting. By creating high-resolution digital images, we can preserve, analyze, and research paintings in ways that were previously impossible.
Authentication
Authentication is a critical component of computer vision in a painting, as it involves the use of computer algorithms to identify forgeries and determine the authenticity of paintings. This is a complex and challenging task, but computer vision algorithms have made significant progress in recent years, and they are now able to achieve high levels of accuracy in detecting forgeries.
There are a number of different techniques that computer vision algorithms can use to authenticate paintings. One common technique is to analyze the brushstrokes in the painting. Forged paintings often have brushstrokes that are inconsistent with the artist’s known style, and computer vision algorithms can be trained to identify these inconsistencies. Another technique is to analyze the color palette used in the painting. Forged paintings often use colors that are not consistent with the artist’s known palette, and computer vision algorithms can be trained to identify these discrepancies.
Computer vision algorithms are also able to identify forgeries by analyzing the painting’s texture. Forged paintings often have a texture that is different from the artist’s known works, and computer vision algorithms can be trained to identify these differences. In addition, computer vision algorithms can be used to analyze the painting’s composition. Forged paintings often have a composition that is different from the artist’s known works, and computer vision algorithms can be trained to identify these differences.
The ability of computer vision algorithms to authenticate paintings has had a significant impact on the art world. In the past, it was often difficult to determine whether a painting was genuine or a forgery. However, computer vision algorithms have now made it much easier to identify forgeries, and this has helped to protect collectors from fraud.
The authentication of paintings is an important component of computer vision in a painting. Computer vision algorithms have made significant progress in recent years, and they are now able to achieve high levels of accuracy in detecting forgeries. This has had a significant impact on the art world, and it has helped to protect collectors from fraud.
Attribution
Attribution is a crucial aspect of computer vision in a painting, as it involves the use of computer algorithms to establish the artist or school responsible for a particular painting. This is a complex task, as it requires computer algorithms to analyze a painting’s visual features and compare them to a database of known artists and schools. However, computer vision algorithms have made significant progress in recent years, and they are now able to achieve high levels of accuracy in attributing paintings.
- Stylistic analysis: Computer vision algorithms can analyze a painting’s brushstrokes, color palette, and composition to identify patterns and characteristics that are consistent with a particular artist or school. For example, computer vision algorithms have been used to identify paintings by Vincent van Gogh, Pablo Picasso, and Leonardo da Vinci with a high degree of accuracy.
- Provenance analysis: Computer vision algorithms can also analyze a painting’s provenance, or history of ownership, to help establish its authenticity. By tracking the painting’s ownership history, computer vision algorithms can identify any suspicious gaps or inconsistencies that may indicate that the painting is a forgery.
- Technical analysis: Computer vision algorithms can also analyze a painting’s technical aspects, such as the type of canvas, the type of paint, and the type of brushstrokes, to help establish its authenticity. By comparing these technical aspects to a database of known works by a particular artist or school, computer vision algorithms can identify any inconsistencies that may indicate that the painting is a forgery.
- Multimodal analysis: Computer vision algorithms can also combine multiple modalities of information, such as visual features, provenance information, and technical information, to establish the artist or school responsible for a particular painting. By combining multiple modalities of information, computer vision algorithms can achieve even higher levels of accuracy in attribution.
The ability of computer vision algorithms to attribute paintings has had a significant impact on the art world. In the past, it was often difficult to determine the artist or school responsible for a particular painting. However, computer vision algorithms have now made it much easier to attribute paintings, and this has helped to shed light on the history of art and to protect collectors from fraud.
Style analysis
Style analysis is a fundamental aspect of computer vision in a painting, as it involves the use of computer algorithms to analyze a painting’s visual features and identify the artistic style of the artist or school responsible for creating it. This is a challenging task, as it requires computer algorithms to be able to recognize and understand the subtle nuances that distinguish one artistic style from another.
-
Brushstroke analysis
Computer vision algorithms can analyze the brushstrokes in a painting to identify patterns and characteristics that are consistent with a particular artist or school. For example, computer vision algorithms have been used to identify paintings by Vincent van Gogh, Pablo Picasso, and Leonardo da Vinci with a high degree of accuracy.
-
Color palette analysis
Computer vision algorithms can also analyze the color palette used in a painting to identify patterns and characteristics that are consistent with a particular artist or school. For example, computer vision algorithms have been used to identify paintings by the Impressionists, who were known for their use of bright, vibrant colors.
-
Composition analysis
Computer vision algorithms can also analyze the composition of a painting to identify patterns and characteristics that are consistent with a particular artist or school. For example, computer vision algorithms have been used to identify paintings by the Renaissance masters, who were known for their use of balanced and harmonious compositions.
-
Multimodal analysis
Computer vision algorithms can also combine multiple modalities of information, such as brushstroke analysis, color palette analysis, and composition analysis, to identify the artistic style of a painting. By combining multiple modalities of information, computer vision algorithms can achieve even higher levels of accuracy in style analysis.
The ability of computer vision algorithms to analyze style is a significant advancement in the field of art history. In the past, it was often difficult to determine the artistic style of a painting, especially if the painting was unsigned or undated. However, computer vision algorithms have now made it much easier to identify the artistic style of a painting, and this has helped to shed light on the history of art and to protect collectors from fraud.
Damage assessment
Damage assessment is an important component of computer vision in a painting, as it involves the use of computer algorithms to detect and analyze damage to paintings for conservation purposes. This is a critical task, as it can help conservators to identify and repair damage before it becomes irreversible.
Computer vision algorithms can analyze a painting’s surface to identify a variety of types of damage, including scratches, tears, punctures, and stains. These algorithms can also be used to measure the extent of damage and to track its progression over time. This information can help conservators to prioritize their work and to develop the most effective conservation strategies.
Here are some real-life examples of how computer vision is being used to assess damage to paintings:
- The Metropolitan Museum of Art in New York City is using computer vision to assess damage to its collection of paintings. The museum’s conservators are using a computer vision system to identify and measure scratches, tears, and other types of damage to the paintings. This information is helping the conservators to prioritize their work and to develop the most effective conservation strategies.
- The Rijksmuseum in Amsterdam is using computer vision to track the progression of damage to its collection of paintings. The museum’s conservators are using a computer vision system to monitor the condition of the paintings over time. This information is helping the conservators to identify paintings that are at risk of further damage and to take steps to prevent it.
The ability of computer vision algorithms to assess damage to paintings is a significant advancement in the field of art conservation. In the past, it was often difficult to detect and measure damage to paintings, especially if the damage was subtle. However, computer vision algorithms have now made it much easier to assess damage to paintings, and this has helped conservators to protect and preserve these valuable works of art.
Hidden details
Computer vision algorithms can be used to uncover hidden details in paintings that are invisible to the naked eye. This is possible because computer vision algorithms can analyze a painting’s surface in much greater detail than the human eye can. Computer vision algorithms can also be used to penetrate the surface of a painting and to visualize the underlying layers of paint. This can reveal hidden sketches, inscriptions, or other details that were previously unknown.
The ability to uncover hidden details in paintings is a significant advancement in the field of art history. In the past, it was often difficult or impossible to see these details without damaging the painting. However, computer vision algorithms now make it possible to uncover these hidden details without causing any damage to the painting.
Here are some real-life examples of how computer vision is being used to uncover hidden details in paintings:
- The Rijksmuseum in Amsterdam used computer vision to uncover a hidden self-portrait of Rembrandt in one of his paintings. The self-portrait was hidden beneath layers of paint and was only revealed after the painting was analyzed using computer vision algorithms.
- The Metropolitan Museum of Art in New York City used computer vision to uncover a hidden sketch of a horse in a painting by Edgar Degas. The sketch was hidden beneath layers of paint and was only revealed after the painting was analyzed using computer vision algorithms.
The ability to uncover hidden details in paintings is a powerful tool for art historians and conservators. This technology can help us to learn more about the creative process of artists and to better understand the history of art.
3D modeling
3D modeling plays a crucial role in computer vision in a painting by providing three-dimensional representations of paintings for immersive experiences and research. This technology allows users to interact with paintings in a more engaging and interactive way, and it also enables researchers to study paintings in greater detail.
One of the most important applications of 3D modeling in computer vision in a painting is the creation of immersive experiences. By creating three-dimensional models of paintings, users can explore the paintings in a virtual environment, zooming in and out to examine details that would be difficult or impossible to see in a traditional two-dimensional representation. This technology is especially useful for studying large or complex paintings, as it allows users to get a better sense of the overall composition and the relationships between different elements.
3D modeling is also a powerful tool for research in computer vision in a painting. By creating three-dimensional models of paintings, researchers can perform a variety of analyses that would be difficult or impossible to do with traditional two-dimensional representations. For example, researchers can use 3D models to study the brushstrokes of a painting, to analyze the composition of a painting, and to identify hidden details that are invisible to the naked eye.
One real-life example of the use of 3D modeling in computer vision in a painting is the work of the Rijksmuseum in Amsterdam. The Rijksmuseum has created a 3D model of Rembrandt’s painting “The Night Watch.” This model allows users to explore the painting in a virtual environment, zooming in and out to examine details that would be difficult or impossible to see in a traditional two-dimensional representation. The model also allows researchers to study the painting in greater detail, and they have used it to identify hidden details that are invisible to the naked eye.The connection between 3D modeling and computer vision in a painting is a powerful one. By creating three-dimensional representations of paintings, computer vision algorithms can be used to analyze paintings in greater detail and to create immersive experiences that allow users to interact with paintings in a more engaging and interactive way.
Examples and Guidelines for “Computer Vision in a Painting”
Computer vision technology offers a wide range of possibilities for analyzing and interpreting paintings. Here are a few examples with guidelines to help you get started:
- Digitizing Paintings: Convert physical paintings into high-resolution digital images using specialized scanning equipment. Ensure proper lighting and color calibration for accurate representation.
- Authenticating Paintings: Analyze brushstrokes, color palettes, and other visual features to identify forgeries. Compare the painting to known works by the artist or period to detect inconsistencies.
- Attributing Paintings: Determine the artist or school responsible for a painting by examining its style, techniques, and provenance. Use computer algorithms to compare the painting to a database of known works.
- Analyzing Artistic Style: Study the brushwork, color choices, and composition to identify the unique characteristics of an artist’s style. This can help distinguish between different artists and periods.
- Assessing Damage: Detect and measure damage, such as scratches, tears, and stains, to aid in conservation efforts. Use computer vision algorithms to quantify the extent of damage and monitor its progression over time.
- Uncovering Hidden Details: Reveal underlying layers, sketches, or inscriptions that are invisible to the naked eye. This can provide insights into the artist’s creative process and uncover previously unknown information.
Tips for Using Computer Vision in a Painting
Tip 1: Choose the Right Tools and Algorithms: Select computer vision techniques that are appropriate for your specific task, such as object detection, image segmentation, or style analysis.
Tip 2: Prepare High-Quality Images: Ensure that the digital images of the paintings are of high resolution and accurately capture the colors and textures.
Tip 3: Train and Validate Models: Develop and train computer vision models using a representative dataset of paintings. Validate the models on a separate dataset to ensure accuracy.
Tip 4: Collaborate with Experts: Consult with art historians, conservators, and other experts to gain insights into the paintings and refine the analysis.
Tip 5: Consider Ethical Implications: Be aware of the ethical implications of using computer vision in a painting, such as the potential for bias or misinterpretation.
Benefits of Computer Vision in a Painting
- Enhanced Art Authentication and Attribution
- Improved Damage Assessment and Conservation
- New Insights into Artistic Techniques and Styles
- Immersive Digital Experiences for Art Appreciation
- Automated Digitization and Archival of Cultural Heritage
By following these guidelines and tips, you can harness the power of computer vision to gain a deeper understanding and appreciation of paintings.
FAQs on Computer Vision in a Painting
Computer vision is revolutionizing the field of art analysis and appreciation. Here are answers to some frequently asked questions about this technology:
Question 1: How does computer vision contribute to art authentication?
Computer vision algorithms analyze visual features of a painting, such as brushstrokes, color palettes, and textures, to identify patterns and characteristics that are consistent with a particular artist or period. This helps experts authenticate paintings and distinguish originals from forgeries.
Question 2: Can computer vision determine the artist of a painting?
Yes, computer vision algorithms can assist in attributing a painting to a specific artist or school. By comparing visual features to a database of known works, algorithms can identify similarities and suggest possible attributions.
Question 3: How is computer vision used to assess damage to paintings?
Computer vision algorithms can detect and measure damage such as scratches, tears, and stains on a painting’s surface. This information aids conservators in prioritizing restoration efforts and developing effective conservation strategies.
Question 4: Can computer vision reveal hidden details in paintings?
Yes, computer vision techniques can uncover underlying layers, sketches, or inscriptions that are invisible to the naked eye. This provides valuable insights into an artist’s creative process and can help uncover previously unknown information about the artwork.
Question 5: How does computer vision enhance the art appreciation experience?
Computer vision enables the creation of immersive digital experiences, allowing users to explore paintings in virtual environments and interact with them in new ways. This enhances art appreciation and makes it more accessible to a wider audience.
Question 6: What are the ethical considerations regarding computer vision in a painting?
It is important to consider the potential for bias or misinterpretation when using computer vision algorithms in art analysis. Collaborating with experts and using diverse datasets can mitigate these concerns and ensure responsible and accurate applications.
In summary, computer vision offers powerful tools for analyzing, authenticating, and preserving paintings. By leveraging this technology, we can gain a deeper understanding and appreciation of our cultural heritage.
Conclusion
Computer vision has revolutionized the study and appreciation of paintings. Through advanced algorithms and image analysis techniques, we can now delve deeper into the world of art, unlocking hidden details, authenticating works, and gaining new insights into artistic styles and techniques. This technology has not only transformed art history but also opened up new avenues for conservation and preservation.
As we continue to harness the power of computer vision in a painting, the possibilities for discovery and innovation are endless. The future holds the promise of even more sophisticated algorithms, enabling us to uncover even more secrets hidden within the canvas. This technology will undoubtedly continue to play a pivotal role in shaping our understanding and appreciation of art for generations to come.
Youtube Video:
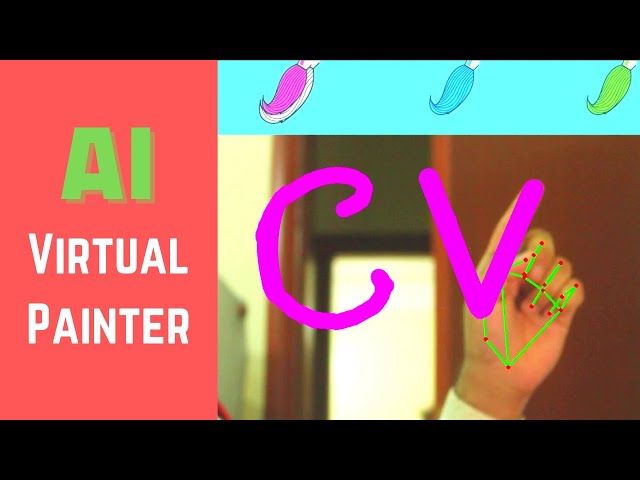